Sepsis is a leading cause of morbidity and mortality. Sepsis is also tough to define and even tougher to predict. Big data and artificial intelligence (AI) promise to make a difference in the diagnosis and prediction of sepsis. But will the combination of massive databases and machine learning actually improve patient outcomes? More importantly, can it?
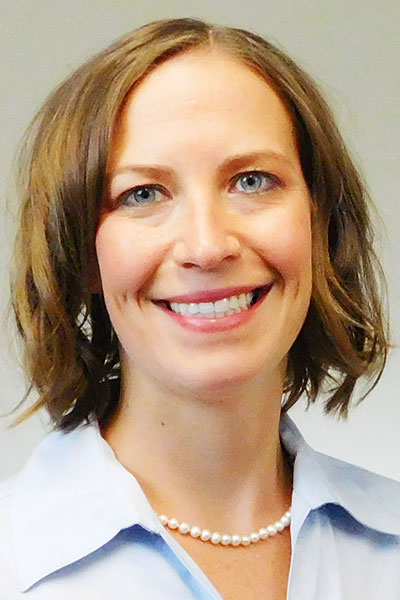
Big Data in Sepsis: From Epidemiology to Diagnosis, on Wednesday, from 8:30 am to 9:30 am, in Room 316B of the convention center, will evaluate the use of sepsis data models during a panel discussion co-chaired by Kathryn Pendleton, MD, FCCP, Assistant Professor of Pulmonary, Allergy, Critical Care and Sleep Medicine at the University of Minnesota, and Past CHEST President, Steven Simpson, MD, FCCP, Professor of Pulmonary, Critical Care, and Sleep Medicine at the University of Kansas Medical Center.
“The real problem is that sepsis is a clinical syndrome,” said Dr. Pendleton, who is also the Director of the Medical Intensive Care Unit at the University of Minnesota Medical Center. “There are things that are highly suggestive of sepsis, but there is no hard and fast, black and white definition. It’s really up to the clinician to think about sepsis and determine what they think the likelihood of this person being septic really is. That’s the problem and the opportunity with AI.”
Over the past decade, many large databases have been analyzed, and major electronic health record systems now incorporate predictive models to better identify current patients and individuals in the ED who present with sepsis. Using AI to better understand those databases and improve predictive models sounds reasonable, Dr. Pendleton said. But sepsis in the real world is a shifting target with fluid definitions.
The current definition of septic shock focuses on end-organ dysfunction as a clinically useful predictor of mortality. That can be a useful definition for clinical trials, Dr. Pendleton said. But clinicians need definitions and help to better identify risk factors predictive of progression to sepsis or septic shock.
“That’s where AI comes in as being helpful,” she said. “These massive databases have all these data points that are changing over time, and AI potentially gives us the ability to build predictive modeling to determine which patients are most at risk for developing sepsis, or are already in the early stages of sepsis, before they exhibit signs of organ failure. The promise is that the model can suggest sepsis as a possibility for the appropriate patients rather than the clinician having to contemplate sepsis de novo on their own. That’s enticing.”
It is also challenging. The most experienced clinicians can’t always distinguish between someone who is at risk for developing sepsis, or is in the early stages of sepsis, from someone who has clinical deterioration or decompensation for other reasons. Not every condition that looks like sepsis actually is sepsis.
Timelines are another challenge.
“Everything we do in sepsis in the current guidelines stems from time zero,” Dr. Pendleton said. “You have, ideally, 1 hour to get fluids, antibiotics, and cultures, maybe 3 hours if you aren’t entirely sure of the diagnosis. But what, really, is time zero? For the emergency department, is it the time the patient hits the door, or does it go back to earliest symptoms? For hospitalized patients, is time zero when they first have a fever? The first low blood pressure reading? The first time lactate is elevated? All of those things happen at different time points, so where do you start counting?
“About 6% of all hospitalizations—more than a third of in-hospital deaths—are due to sepsis,” she said. “These are the kinds of questions we need to deal with if we are going to reduce that toll.”